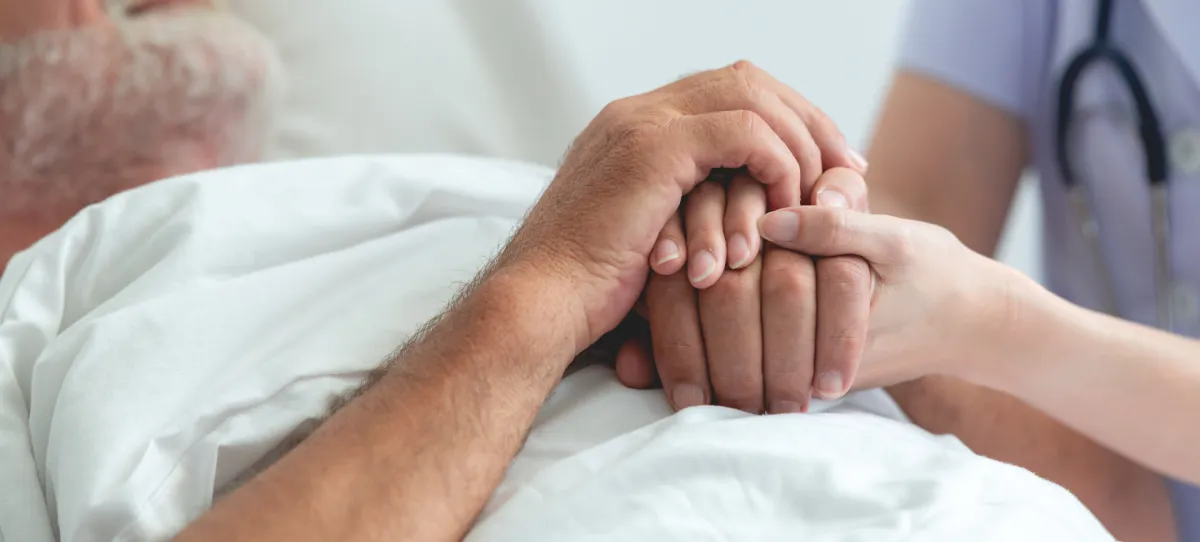
Provider Spotlight: Predictive Models Help Plan for End-of-Life-Care with a Health Equity Lens
Dr. Susan Beane, Executive Medical Director, Healthfirst
Vincent Major, Ph.D., Assistant Professor of Population Health, New York University Grossman School of Medicine
Introduction by Dr. Susan Beane
In 2019, a study revealed that, for the first time in nearly a century, the home was the most common place of death in the United States. Nearly 31% of Americans who died in 2017 did so at home, compared to just under 30% who died in the hospital and nearly 21% in nursing homes.
This is a welcome development: Polls have shown that more than 70% of Americans prefer to die at home.
However, the same study showed racial and ethnic minorities had lower odds of dying at home than White patients. There’s still a clear disconnect between patients’ desire and what occurs.
One reason for this disconnect is that physicians don’t know a patient’s plans for end-of-life care. Sometimes, a patient hasn’t had this conversation with their loved ones — which is understandable, as end-of-life care is expensive and complex, not to mention stressful and quite frankly, not easy. In other cases, the talk has happened, but their wishes haven’t been documented anywhere that a physician can see it.
Vincent Major, Ph.D., is an assistant professor of population health at New York University’s Grossman School of Medicine. He’s also an affiliate of the organization’s Predictive Analytics Unit, which aims to ensure artificial intelligence models can be incorporated into everyday practice. One of the models he and his colleagues have developed is a predictor of mortality risk viewed through the lens of end-of-life planning, which helps health system providers have more conversations about advance care planning. A critical component of this work has been learning how to adjust the model’s use to balance equality and equity in care.
Encouraging More Conversations About Advance Care Planning
By Vincent Major, Ph.D., Assistant Professor of Population Health, New York University Grossman School of Medicine
While every patient has a different trajectory with chronic illness, many experience something called a sentinel hospitalization. This is a moment when a patient’s condition worsens to the point that it’s necessary to reassess their prognosis, treatment options, and the goals of their care.
A sentinel hospitalization isn’t difficult to pinpoint in retrospect. Anyone who has lost a loved one can recall a moment when things took a turn and, maybe, the conversations with the care team began to shift from aiding recovery to providing comfort. However, it’s often quite difficult to acknowledge this shift in the moment.
Our goal with the predictive model for mortality was to identify patients who had been admitted to the hospital and were at high risk of dying within 60 days. We wanted to run this model within minutes of the order to admit being issued so providers could do two things: contextualize all of their care decisions with awareness of the patient’s prognosis and have, as well as document, a conversation about advance care planning with the patient and their loved ones.
We built the model using our own EHR data — 128,000 admissions over three years — and a data set we acquired that includes deaths derived from the Social Security Administration. Since implementing these models, we’ve seen an increase in the number of documented Advance Care Plans (ACPs) for some of our most at-risk patients. Our system helps to ensure that our patients are consulted on their end-of-life care wishes and helps our physicians to be better equipped to provide care that aligns with those wishes. We’ve also been applying this model to screen patients for a care management process where advance care planning is incorporated into conversations with community health workers or other care coordinators once a patient has been discharged to their home.
Adjusting the model’s use to address its disparities
One thing we noticed as the mortality prediction models were put to use was a disparity in their outcomes. We were running the same models at multiple campuses, but the patients being treated at one location were less likely to surpass the model’s cutoff and be guided to the ACP conversation.
We discovered this was a result of the distribution of data. Our model is built on the presumption that utilization is proportional to risk, as more visits to doctors, the emergency department, or the hospital are indicative of a greater risk of dying. However, patients with limited access to care at that location — or, correspondingly, more appointments from providers outside our system — would have less data against which the predictive model could run. Because their utilization needs weren’t part of the data set, the model could unfairly downplay their risk.
This left us facing a critical question. Would we use the model to provide equal care — and continue to disadvantage the patients at that location — or would we change the way we use the model to provide more equitable care and ensure that resources go to the patients who need them?
Our health system opted to develop different versions of the predictive model depending on the data that we have available on a patient. That way, if we have a newly registered patient, who has no existing data in our EHR system, we can run a “sibling” model using only the content of their history and physical examination note, helping to level the playing field for all types of patients.
Another part of our analysis has been to explore changes to the model’s threshold for patients admitted at different hospital locations. That way, we could use the same predictive model across the organization but modify the threshold as a correction factor to help ensure more patients who may benefit from ACP conversations can receive the recommended intervention. Several factors complicated this approach including how both patient outcomes and access to care have been improving over time, as well as the introduced unfairness when a single patient would be treated differently depending on which hospital they happen to arrive to.
In addressing this issue, we learned an important lesson about bias in AI. We discovered that dismissing bias wasn’t helpful. Instead, we saw the limitation that it presented to us as an opportunity to address existing disparities and determine paths for correcting them. Awareness of this health equity issue incentivized us to provide alternative pathways to discover at-risk patients so that we could expand our program and discover more, and complementary types of, patients who could benefit from end-of-life planning.