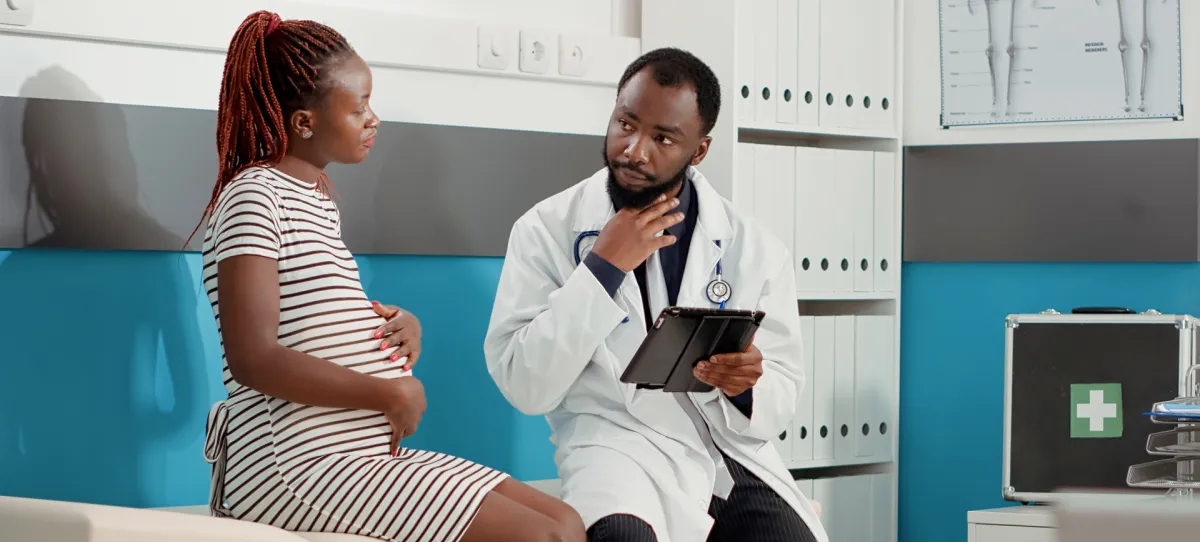
High-Risk Pregnancy Intervention
Using Predictive Modeling to Identifying High-Risk Pregnancies to Prevent Avoidable Complications in Populations at Greater Risk for Maternal and Infant Mortality
Dr. Susan Beane, Executive Medical Director, Healthfirst
Jennifer Zbell, Vice President, Clinical Analytics
Sule Baptiste, Director of Data Science, Healthfirst
Healthfirst is committed to providing equitable care for all New Yorkers. Pregnancy care and family planning is a core component of this mission.
Variations in care quality, underlying chronic conditions, implicit bias in the practice of medicine, and structural racism in maternity care contribute to maternal mortality rates that are five times higher for Black women than White women in New York State and three times higher nationally.
Once born, Black infants in New York City are 50% more likely to have unexpected complications, and Hispanic infants are 20% more likely. This is partly due to the fact that these infants are more likely to be delivered in hospitals with high-rates of complications.
One important strategy for reversing this trend is identifying those who are at a high-risk of a complicated pregnancy and intervening sooner to avoid preterm delivery, stays in the neonatal intensive care unit (NICU) or other complications. Healthfirst’s data analytics team is working to build machine learning frameworks to predict risk and empower care teams to act.
Identifying risk based on hundreds of factors
Within maternity care, there are already many decision trees or business rules that help to identify whether someone is at high-risk of a complicated pregnancy. Factors such as age, weight, characteristics of the cervix and pregnancy history can be used to risk stratify pregnant individuals.
The beauty of machine learning is that it can aggregate multiple decision trees. Instead of evaluating risk based on a handful of factors, we can build predictive models that examine more than 300 data points. These primarily come from claims, but we also have data on individual members and providers, and we supplement this with public health data when we can.
We can also incorporate factors that often get overlooked. One example is patterns in healthcare utilization. Does someone have a relationship with a primary care physician and any relevant specialists? How frequently are they utilizing services such as telehealth, urgent care, or behavioral health? What is their history of refilling prescriptions?
As you may expect, most pregnant individuals are low risk, and risk increases as the population is divided into percentiles. At the 90th percentile, a high-risk delivery is more than twice as likely; for the 99th percentile, it’s about four times as likely.
Intervening seven weeks earlier
When we incorporated this predictive model into our Healthfirst Cares Maternity Program, we were able to identify high-risk members 52 days (or more than seven weeks) earlier than traditional clinical decision trees.
Given that the average pregnancy is 280 days (or roughly 40 weeks), that’s significant. We don’t have to wait until a glucose screening (usually done between 24 or 28 weeks) or an anomaly scan (usually between 18 and 22 weeks) to identify the presence of gestational diabetes or congenital defects, respectively. Our model can flag risk factors weeks earlier, and we can intervene in ways that will positively impact the health of parent and child.
In one case, we had a member who was having her third baby. The first two had been admitted to the NICU due to pre-term deliveries complicated by gestational diabetes, and she was presenting with insulin-dependent gestational diabetes with her third baby.
The predictive model identified this member, and after she spoke to a care management nurse, she accepted the offer of a referral to a nutritionist. With the nutritionist’s help, she lost weight, was able to discontinue her insulin, and delivered at full term without a NICU admission.
While this member’s story points to the importance of machine learning as a screening tool, it’s also important to note the steps that follow member identification. Care teams need a mechanism to recommend and provide the right resources at the right time. It doesn’t necessarily matter who delivers the care — primary care, specialty care, care management, home health, social services, and so on — as long as the member gets the care they need and avoids preventable and costly complications and mom and baby are healthy.